Meta-analysis is a widely accepted and collaborative method to synthesize research findings across various disciplines (Cheung & Vijayakumar, 2016). It is a fundamental tool that combines outcome data from individual trials to produce pooled effect estimates for different outcomes of interest. This process increases the sample size, improves the statistical power of the findings, and enhances the precision of the effect estimates. Synthesizing results across studies is crucial for understanding a problem and identifying sources of variation in outcomes, making it an essential part of the scientific process (Gurevitch et al., 2018). The reliability of the information presented relies on the calibre of the studies included and the thoroughness of the meta-analytical procedure. Some concerns have been expressed about the ultimate usefulness of such a complex and time-consuming procedure in establishing timely, valid evidence on various specified topics throughout the evolution of the current meta-analytic methodology (Papakostidis & Giannoudis, 2023).
Meta-analysis is a robust method for consolidating data from multiple studies to generate evidence on a specific topic. It is a statistical technique used to combine the findings of several studies (Gurevitch et al., 2018). However, there are various crucial considerations when interpreting the results of a meta-analysis.
Meta-analysis is a scientific research approach that objectively evaluates the literature on a given subject. As a collection of statistical methods for aggregating the effect sizes across different datasets addressing the same research question, meta-analysis provides a potent, informative, and unbiased set of tools for summarizing study results on the same topic. It offers several advantages over narrative reviews, vote counting, and combining probabilities (Table 1.). Meta-analysis is based on expressing the outcome of each study on a standard scale. This "effect size" outcome measure includes information on each study's sign and magnitude of an effect of interest. In many cases, the variance of this effect size can also be calculated (Koricheva et al., 2013).
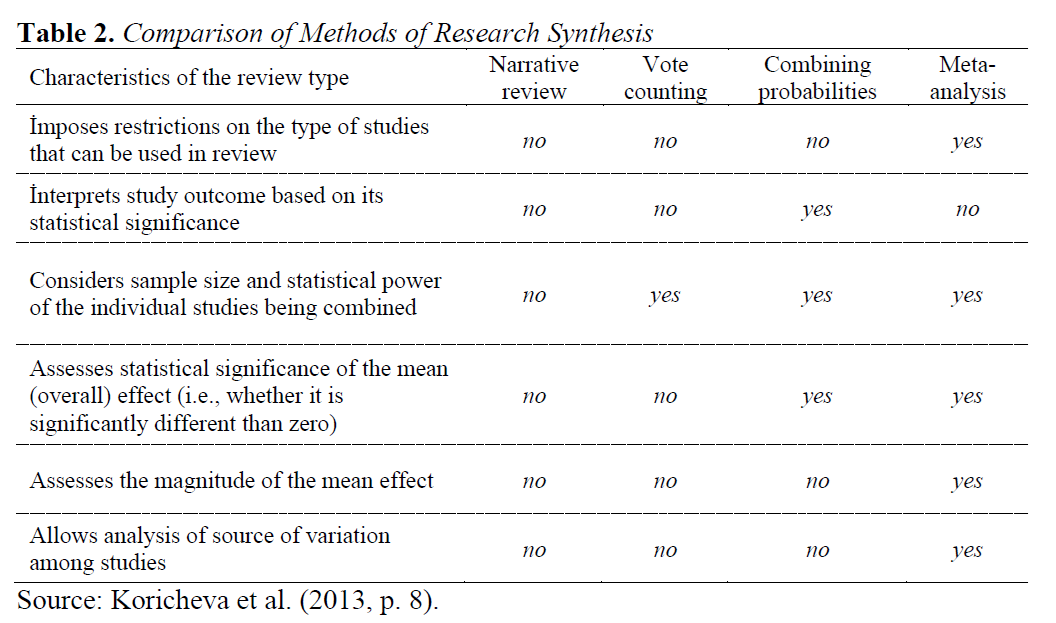
Meta-analysis involves combining the findings of several studies to estimate a population parameter, usually an effect size, by calculating point and interval estimates. In addition, meta-analyses are important for identifying gaps in the literature, highlighting areas where more research is needed and areas where the answer is definitive, and no new studies of the same type are necessary. This aspect of meta-analysis helps keep the audience informed about the research landscape, guiding them towards areas that require further exploration.
Meta-analyses are fundamental tools of Evidence-Based Medicine (EBM) that synthesize outcome data from individual trials to produce pooled effect estimates for various outcomes of interest. Combining summary data from several studies increases the sample size, improving the statistical power and precision of the obtained effect estimates. Meta-analyses are considered to provide the best evidence to support clinical practice guidelines. The quality of the evidence presented relies on the calibre of the studies included and the thoroughness of the meta-analytic procedure. Some concerns about the usefulness of such a complex and time-consuming procedure in establishing timely, valid evidence on various specified topics have been expressed.
A systematic review is a consistent and reproducible qualitative process of identifying and appraising all relevant literature to a specific question. Meta-analysis takes this process further by using specific statistical techniques that allow for a quantitative pooling of data from studies identified through the systematic review process.
A meta-analysis can be carried out if the systematic review uncovers enough and suitable quantitative information from the summarised studies (Gurevitch et al., 2018).
Meta-analysis is now a popular statistical technique for synthesizing research findings in many disciplines, including educational, social, and medical sciences (Cheung, 2015). Google Scholar published over 107,000 meta-analyses in 2022 alone (Irsova et al., 2023). Classical meta-analysis is aggregated person data meta-analysis, in which multiple studies are the analysis units. Compared to the original studies, the analysis of multiple studies has more power and reduces uncertainty. Following this, different meta-analysis approaches have been developed. Therefore, with prior knowledge of the differences between these approaches, it is clear which approach should be used for the data aggregation. For example, in the early days, different meta-analytic approaches used the aggregation of different types of effect sizes (e.g., d, r); today, the transformation of effect sizes is common (Kaufmann & Reips, 2024).
It's important to note that meta-analysis has two distinct aggregation models: the fixed and the random effects model. The fixed effects model operates under the assumption that all studies in the meta-analysis stem from the same population and that the true magnitude of an effect remains consistent across all studies. Therefore, any variance in the effect size is believed to result from differences within each study, such as sampling errors.
Unlike the fixed-effects model, the random-effects model supposes that effects on the population differ from one study to another.
The idea behind this assumption is that the observed studies are samples drawn from a universe of studies. Random effects models have two sources of variation in a given effect size: variation arising from within studies and from variation between studies.
Evidence from a meta-analysis is inherently associated with the quality of the primary studies. Meta-analyses based on low-quality primary studies tend to overestimate the treatment effect.
Consider this: Why should we conduct a meta-analysis instead of relying solely on leading experts' reviews or primary single-study investigations as sources of the best evidence? This question prompts us to delve deeper into the unique benefits and insights that meta-analysis can offer.
While meta-analysis presents numerous benefits, including increased precision, the ability to address new questions, and resolving conflicting claims, it's crucial to tread carefully. If not conducted with meticulous attention, meta-analyses can lead to misinterpretations, mainly if study designs, biases, variation across studies, and reporting biases are not thoroughly considered (Higgins et al., 2023).
Understanding the type of data resulting from measuring an outcome in a study and selecting appropriate effect measures for comparing intervention groups is of the utmost importance. Most meta-analysis methods involve a weighted average of effect estimates from different studies, a decision that rests on the researcher's shoulders.
Studies with no events provide no information about the risk or odds ratios. The Peto method is considered less biased and more powerful for rare events. Heterogeneity across studies must be considered, although many reviews do not have enough studies to investigate its causes reliably. Random-effects meta-analyses address variability by assuming that the underlying effects are normally distributed, but it is essential to interpret their findings cautiously. Prediction intervals, which are a range of values that are likely to include the true effect, from random-effects meta-analyses help illustrate the extent of between-study variation.
Preparing a meta-analysis involves making numerous judgments. Among these, sensitivity analyses stand out as a crucial tool. They should meticulously examine whether overall findings are robust to potentially influential decisions, providing a reassuring layer of reliability and robustness to your research.
Preparing a meta-analysis requires many judgments. Sensitivity analyses, a crucial tool, should examine whether overall findings are robust to potentially influential decisions, ensuring the reliability and robustness of your research (Deeks et al., 2023).
Many leading journals feature review articles penned by experts on specific topics. While these narrative reviews are highly informative and comprehensive, they express the subjective views of the author(s), who may selectively use the literature to support personal views. Consequently, they are susceptible to numerous sources of bias, relegating them to the bottom of the level-of-evidence hierarchy. This underscores the critical importance of conducting high-quality meta-analyses, which can provide a more objective and comprehensive view of the available evidence.
Systematic reviews and meta-analyses are meticulously designed to minimize bias in a marked departure from narrative reviews. They achieve this by identifying, appraising, and synthesizing all relevant literature using a transparent and reproducible methodology. This rigorous approach ensures that the evidence obtained is the most reliable, establishing systematic reviews and meta-analyses as the gold standard at the pinnacle of the hierarchy of evidence.
However, given the massive production of flawed and unreliable synthesized evidence, a major overhaul is required to generate future meta-analyses. The quality of the chosen studies should receive strong attention, as should the consistency and transparency in conducting and reporting the meta-analysis process.
Conducting a meta-analysis properly involves combining data from multiple individual studies, ideally randomized control trials, to calculate combined effect estimates for different outcomes of interest. This is particularly useful for reconciling conflicting results from the primary studies and obtaining a single pooled effect estimate that is thought to represent the best current evidence for clinical practice. Moreover, through significantly expanding the sample size, meta-analyses enhance the statistical strength of their results and, ultimately, offer more accurate effect assessments.
Meta-analyses can be classified as cumulative/retrospective or prospective. The predominant approach in the literature is cumulative. However, in a prospective meta-analysis (PMA), study selection criteria, hypotheses, and analyses are established before the results from studies pertaining to the PMA research question are available. This approach reduces many issues associated with a traditional (retrospective) meta-analysis (Seidler et al., 2019).
The results of a meta-analysis are presented graphically in a forest plot (see Fig. 5). A forest plot would display the effect size estimates and confidence intervals for every study included in the meta-analysis. The meta-analysis should also assess the heterogeneity of the included studies. Commonly, heterogeneity is assessed using statistical tests. The x2 and I2 tests are widely used. A x2 test with a P-value of > 0.05 or I2 greater than 75% indicates significant heterogeneity. In conducting a meta-analysis, you can utilize either a fixed effect model or a random effect model. If there is no heterogeneity, a fixed effect model is used; otherwise, a random effect model is applied. An assessment of publication bias is also required to check that positive, significant, or small studies do not influence the results. Results are displayed graphically in a funnel plot (see Fig. 5), recommended where more than ten studies have been included in the meta-analysis (Yusuff, 2023).
Despite the ongoing methodological deficits in currently published meta-analyses, there is a clear path to improvement. When conducted in adherence to strict and transparent rules, systematic reviews and meta-analyses can ensure the reproducibility and robustness of the search process, the reliability and validity of their findings, and the clarity of reporting.
The meta-analysis process involves a thorough approach, considering all potential influences on the results. For example, the random-effects model assumes that the true effect estimate varies among the primary studies due to differences in their clinical characteristics. This model's combined effect size estimate represents an average estimate of all the individual study estimates. Choosing the correct statistical model for combining data is a complex decision that hinges on the degree of variation between studies. However, there are no clear thresholds regarding the amount of variation that would determine which model to use.
Moreover, the statistical tests for variation often need more power to detect significant differences. The fixed-effects model is generally used when there is no variation in a meta-analysis, especially when many studies with large sample sizes are included. In such cases, there is confidence in the ability of the variation test to detect significant differences. Results from this model usually have narrower confidence intervals. On the other hand, when there are concerns about variation, the random-effects model is considered a better choice. It generates wider confidence intervals around the estimates and is a more conservative option for the analysis. In a meta-analysis with many studies and adequate sample sizes, where statistical variation is not detected, using the fixed-effects model is justified (Papakostidis & Giannoudis, 2023).
Finally, the quality of evidence obtained through a meta-analysis should be evaluated using one of three tools: GRADE (Grading of Recommendations Assessment, Development and Evaluation)*, PRISMA (Preferred Reporting Items for Systematic Reviews and Meta-Analysis)** or AMSTAR (A Measurement Tool to Assess systematic Reviews)***. All these tools assess confidence in the effect estimate for each specific outcome of interest. Its use significantly enhances the strength and dependability of the findings, offering researchers assurance about the quality of their research. Therefore, they are a crucial component of meta-analysis that should be considered.
Even though meta-analyses, particularly those based on high-quality RCTs, are regarded to provide the best evidence, the problem of inconclusiveness of a meta-analysis is not associated with a potentially diminished methodological quality or lack of adherence to the accepted standards of conducting and reporting a proper meta-analysis. The problem is that most systematic reviews are flawed, misleading, redundant, useless or all of the above (Ioannidis, 2017).
Papakostidis and Giannoudis (2023) point out that innovative types of systematic reviews and meta-analyses (some of them stemming from older ideas) are likely to witness a renowned interest soon to achieve a more reliable evidence synthesis. There are four types of such innovative meta-analyses:
- Prospective meta-analysis, a method based on designing prospective trials with a predefined purpose, offers a promising approach. When these trials are completed, they can serve as primary studies for a meta-analysis. This method can address a wide range of research questions, from focused clinical inquiries to comprehensive research agendas, demonstrating its versatility and potential impact. This adaptability can inspire the audience about this method's wide range of applications.
- Meta-analysis of individual participants' data, while offering a more robust approach to handling confounders and formulating new hypotheses, presents its challenges. These include potential time constraints and logistical complexities. Moreover, the risk of selective reporting bias should be seriously considered, underscoring the need for meticulous planning and execution. This awareness of the challenges can make the audience feel prepared and cautious.
- Network meta-analyses allow the analytic process to be extended to more than two treatment groups, utilizing direct and indirect comparisons between them. This approach not only provides a more comprehensive understanding of the treatment landscape but also allows for the comparison of treatments that have not been directly compared in individual studies. Although most are based on already published data, they can still build on prospective meta-analytic designs or individual-level data.
- Umbrella meta-analyses, which synthesize evidence from all relevant systematic reviews and meta-analyses on a specific topic, constitute an attractive way to distil and translate large amounts of evidence.
* https://www.gradeworkinggroup.org/
** https://www.prisma-statement.org/
*** https://amstar.ca/index.php