The experimental study design has the most significant level of control. It has frequently been identified as the gold standard of quantitative research due to its ability to determine a cause-and-effect relationship between an intervention (the cause) and the study outcome (the effect) (Rogers & Révész, 2020).
Scientific research widely recognises experimental designs as the gold standard. This method, known as true experimentation, establishes a cause-and-effect relationship between variables within a study. Despite common misconceptions, true experimentation is not exclusive to laboratory settings.
Experimental research provides a structured approach to establishing causal relationships between variables. Using this approach, the researcher is actively involved in deducing and testing hypotheses. The researcher manipulates an independent variable (cause) and observes its effect on a dependent variable while attempting to control for extraneous variables. This is achieved by administering the treatment to one group while withholding it from another and then analysing the resulting scores of both groups.
In the realm of research, an experiment involves selecting participants randomly and exposing them to different levels of one or more variables, known as independent variables. The researcher then observes the impact of this exposure on one or more outcome variables called dependent variables. The aim of conducting an experiment is to establish a correlation between the independent and dependent variables and deduce conclusions regarding the effectiveness of the intervention and its causal connection. A key aspect of this process is controlling for extraneous variables. This control is essential as it ensures that any observed effects are solely due to manipulating the independent variables, enhancing the study's validity. Experiments are a potent tool for investigating cause-and-effect relationships in diverse fields, including psychology, medicine, physics, and engineering (Mizik & Hanssens, 2018).
True experiments randomly allocate subjects to treatment conditions, while quasi-experiments utilise nonrandomised assignments. To ensure the similarity of subjects, cases are matched on various characteristics and randomly assigned to control and experimental groups. Only observable facts are considered, and inferential statistics produce precise numerical results. Different experimental designs have been developed, from simple before-after to complex multivariate factorial designs, including:
- Parallel design - In a parallel design, participants are randomly assigned to either the intervention or control group.
- Crossover design - In a crossover design, participants are initially assigned to either the intervention or control group and then switch over to the other group after a certain period of time. This design helps negate bias from individual differences since each participant becomes their own control.
- Cluster design - In many research contexts, it is not always possible to randomise individuals to receive different interventions. To overcome this, groups or clusters of individuals (for example, wards, units or hospitals) can be randomly assigned to either the control or intervention, and all cluster members will receive the allocation.
The randomised controlled trial (RCT) is a highly valued research approach that embodies these virtues (Styles & Torgerson, 2018) (Fig. 11).
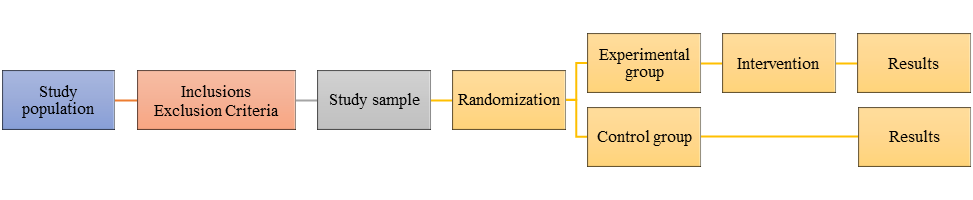
Figure 11. RTC-Randomised Controlled Trial (Crano et al., 2014).
In this type of experiment, all variables are identified and controlled except for one. The independent variable is manipulated to observe its effects on dependent variables. Additionally, participants are randomly assigned to experimental treatments instead of being selected from naturally occurring groups, which ensures the validity of the research.
The fundamental principles of experimental designs include random assignment, variable manipulation, and control groups. While experimental designs effectively establish causal relationships, they have limitations, such as ethical considerations and practical constraints.
The fundamental framework of a quantitative design is rooted in the scientific method, utilising deductive reasoning. This involves the researcher developing a hypothesis, investigating to gather data on the problem, and then analysing and sharing the conclusions to demonstrate that the hypotheses are not untrue.
To follow this procedure, one should:
- Observe an unknown, unexplained, or new phenomenon and research the current theories relating to the issue.
- Create a hypothesis to explain the observations made.
- Predict outcomes based on these hypotheses and create a plan to test the prediction.
- Collect and process data. If the prediction is accurate, proceed to the next step. If not, create a new hypothesis based on the available knowledge.
- Verify the findings, draw your conclusions, and present the results in a suitable format.
Crano et al. (2014) outline the steps of a classic true experimental research design, which involve gathering a group of participants, conducting a pre-test on the dependent variable, randomly assigning participants to either the experimental or control group, closely controlling the application of the experimental treatment between the two groups, and measuring both groups again on the dependent variable after the experimental manipulation (Fig. 12). Variations exist, such as removing pre-testing, including multiple experimental treatments, or using the same participants across all experimental conditions.
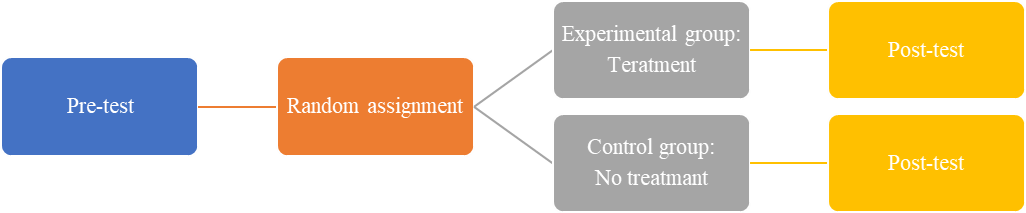
Figure 12. Pre-test - Post-test Control Group Design (Crano et al., 2014).
Following crucial steps is essential to designing and conducting experiments effectively. These steps include the following (Kuçuksayraç, 2007):
- Sampling participants for the study.
- Randomly assigning participants to groups.
- Randomly assigning groups to experimental or control conditions.
- Defining the independent variable, which refers to the aspect of the environment being studied that varies between the groups.
- Defining the dependent variable, which measures any resulting behavioural changes.
- Controlling all other variables that may affect the dependent variable while keeping the independent variable consistent.
- Conducting statistical tests to confirm or refute the hypothesis to determine whether there are any differences between the two groups regarding the dependent variable measurements.
- If the hypothesis is confirmed, explain and generalise the findings.
- Finally, predict how the findings may apply to other situations, potentially through replication.
In conclusion, quantitative research is an essential method for measuring variables and evaluating the effectiveness of interventions. Unlike qualitative research, quantitative research is conducted objectively, emphasising reducing bias. Researchers who seek to adopt evidence-based practices must have a strong understanding of quantitative research design. This knowledge allows them to comprehend and evaluate research literature better and potentially integrate study outcomes and recommendations into their work.
Table 8. summarises the alternatives for implementing quantitative and experimental research projects adjusted to different research conditions.
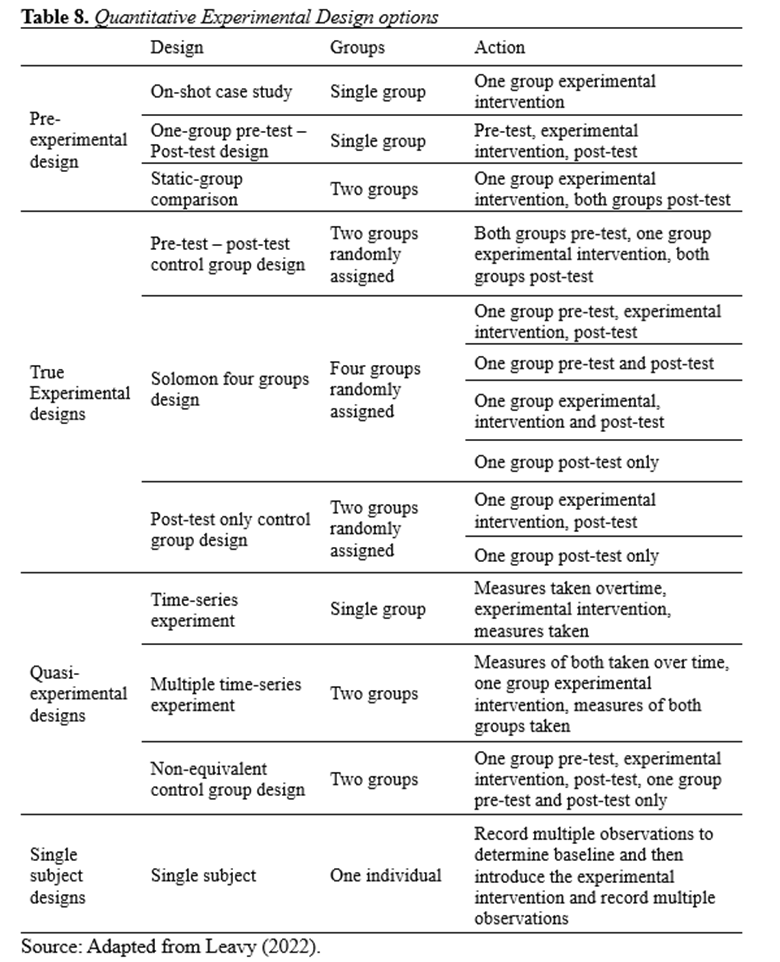